We now live in the era of AI, ML, and public web data. The power of data to drive innovation, decision-making, and competitive advantage across several sectors is comparable to that of oil in our period. In publicly available online data, there is a treasure trove of usable knowledge, including firmographics, personnel statistics, job ads, financing insights, and much more. Merging artificial intelligence (AI) and machine learning (ML) with publicly available online data is not some far-off concept. Rather, it is quickly becoming a reality, according to industry forecasts that predict the big data analytics market will reach $745.15 billion by 2030.
The Untapped Potential in the AI, ML, and Public Web Data Era
Public web data plays an important role in this rapid expansion, with unrealized transformational potential. But until more sophisticated tools come along, a lot of this data is sitting dormant, ready to be unleashed. The data-processing powerhouses of AI and ML can do the following:
-
- Sift through this mountain of information
-
- Transform raw data into insights that can guide strategy
-
- Propel innovation
-
- Give companies a leg up in the marketplace
We still don’t know how fast industries can change to make good use of this massive data pool. Therefore, we don’t know what opportunities lie ahead.
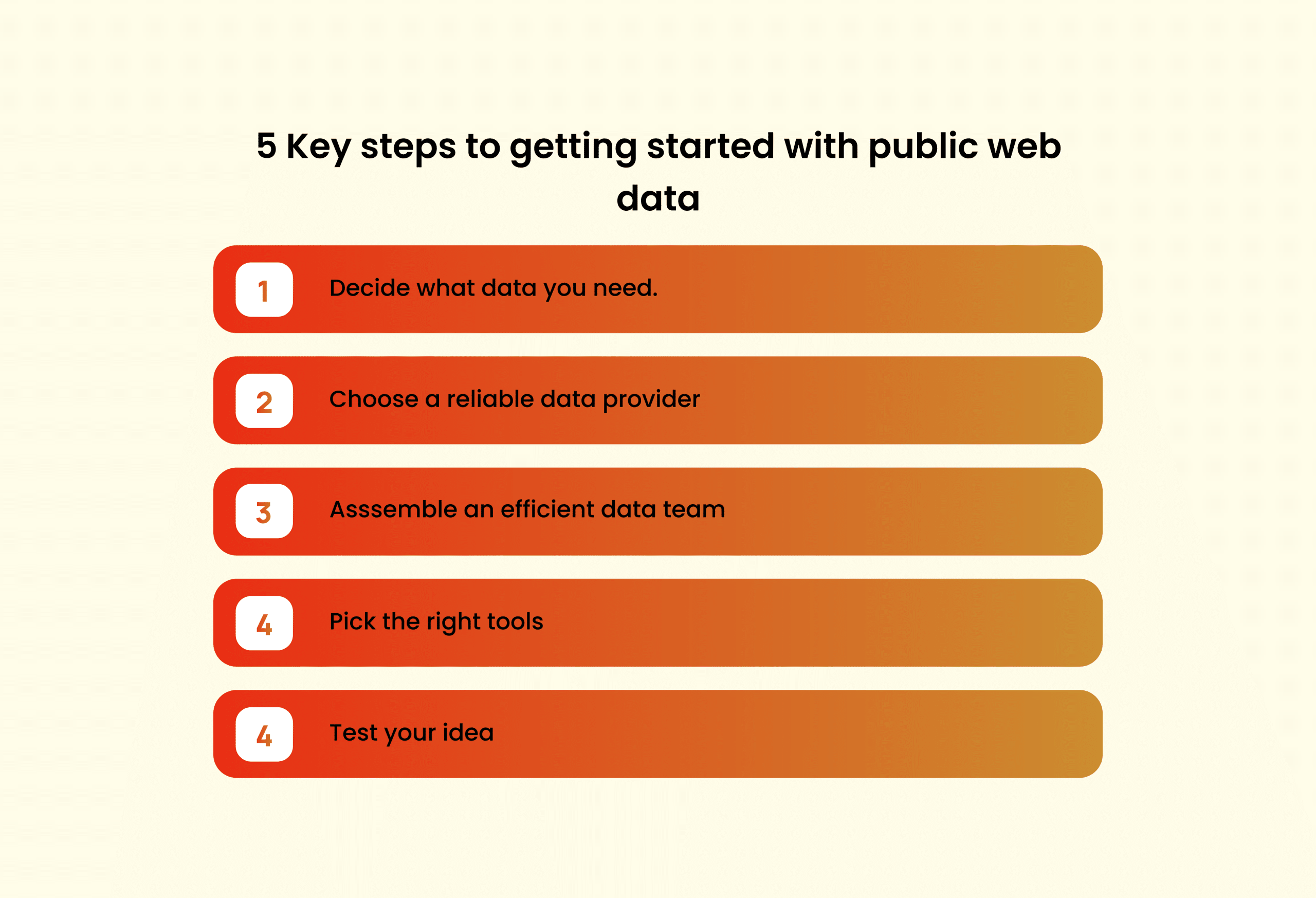
Follow our blog for more insightful posts.
The Age of Fusion
In today’s technological and economic worlds, AI and ML have had an indisputable influence. For large-scale tailored experience delivery, data processing, and the development of predictive analytics models, these technologies are now indispensable.
So, how precisely is this degree of productivity and creativity attained? Combining AI and ML with publicly available web data is a key component of the solution. Public web data, AI, and ML are not only complementary; they are inseparable. It’s a huge step forward that will change the way companies work, come up with new ideas, and compete. The chance to develop AI applications that are intelligent, responsive, and complex has never been higher than it is now, thanks to the abundance of data.
Spectrum of Applications
New paradigms of operational efficiency and strategic innovation are being crafted in this exciting age of technological growth via the interaction of AI, ML, and public web data.
1. Improved decision-making
By analyzing large amounts of diverse public data, AI and ML systems reveal trends and patterns that humans might miss.
2. Enhancing marketing and sales efforts
The goal is to synchronize the delivery of relevant messages to specific audiences using the intelligent analysis of publicly available web data.
3. Potential investment possibilities
Machine learning and artificial intelligence sift through mountains of data in search of patterns in investment, new business formation, and potential industries.
4. Updates to HR software
With the use of artificial intelligence and LLM (large language models), human resources departments may scour publicly available online data for qualified applicants who fit the bill and would fit in well with the company’s culture.
With ML’s assistance, you can sift through reams of applicant profiles and extract useful context from their CVs. Looking for certain keywords in resumes is common. Nevertheless, it might cause you to miss out on a lot of qualified applicants who only used alternative terms to explain their background and abilities. Machine learning, and LLM in particular, is useful in these situations.
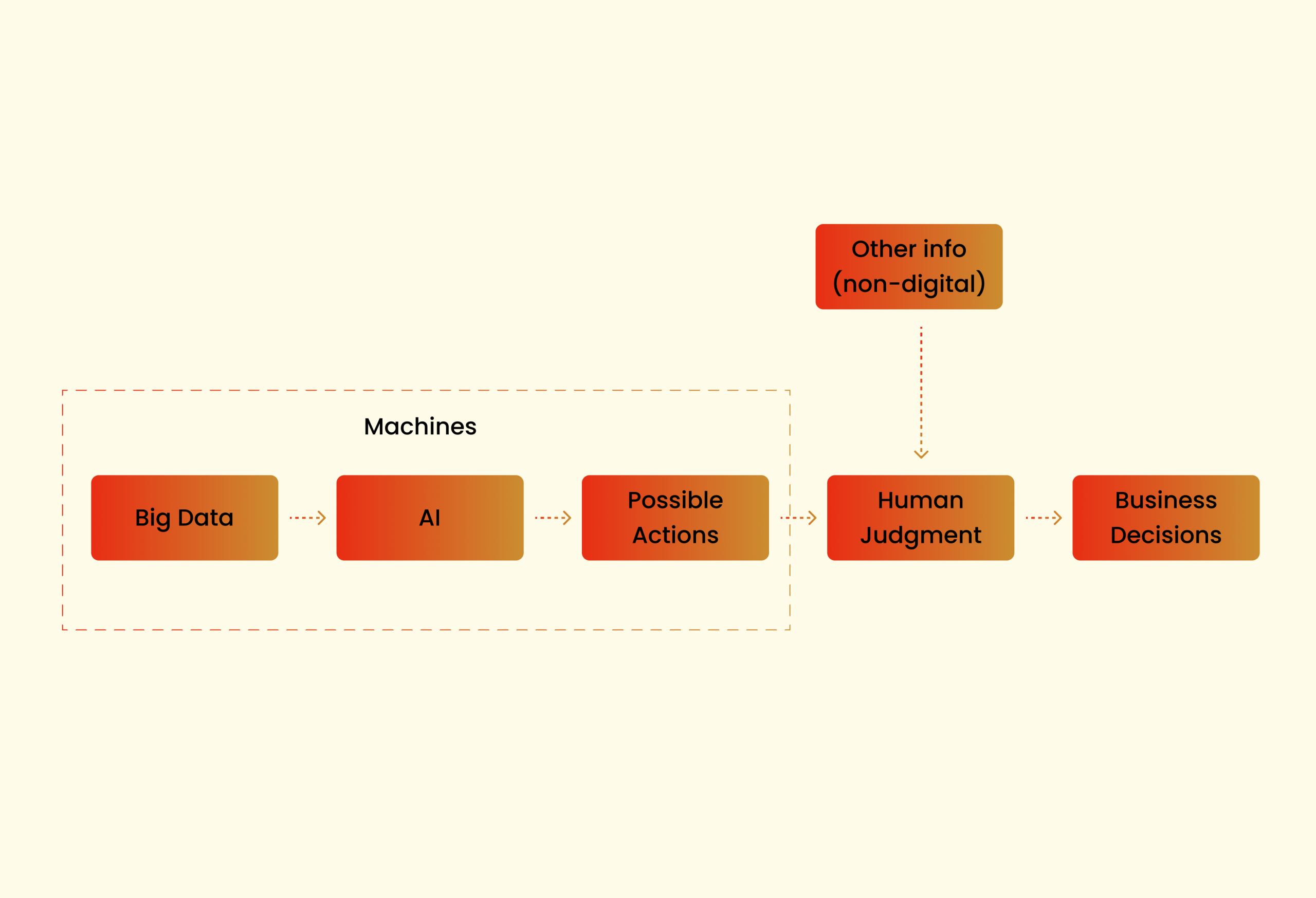
Obstacles to Confront
While there is a great deal of room for development and innovation, there are also significant obstacles, particularly with regard to data accuracy and privacy.
1. Problems with copyright, privacy, and the law
This discussion must prioritize issues of copyright, privacy, and other legal natures. In order to respect the rights of individuals and organizations, we need to be very careful when we collect and use data from the public web. An important step forward has been the litigation against Google for data scraping. This has brought attention to the sometimes complicated and convoluted ethical and legal factors.
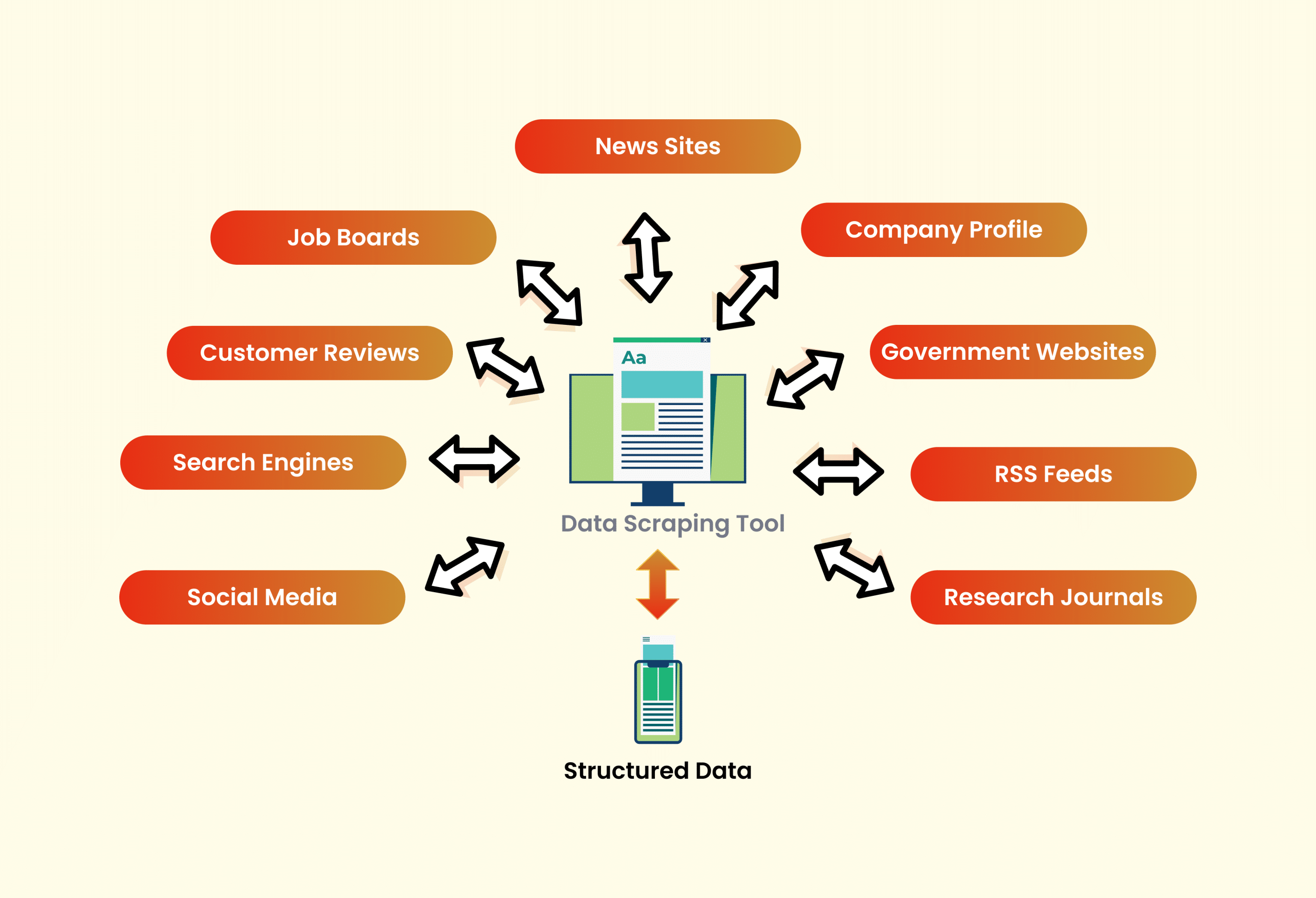
2. Accuracy
Another crucial component is the precision of the data. In order to get useful insights from the vast and varied public online data, it is essential to verify its accuracy and relevancy. Inaccurate or out-of-date data poses a threat to the reliability of analytics and forecasts. This, in turn, may cause poor decision-making. The reliability and efficacy of AI and ML systems are inextricably bound up with the caliber of the data they handle.
3. Ethics
Beyond issues of accuracy and privacy, there are further ethical concerns to bear in mind. There is an examination of the methods used to gather, examine, and use data. Building confidence and trust in AI and ML applications relies on data extraction that follows ethical standards. Consequently, how can we responsibly and precisely traverse these waters? It all starts with implementing stringent data governance rules.
4. Collaboration
Furthermore, a cooperative strategy including regulatory agencies, IT firms, and other interested parties may cultivate a climate of collective accountability. For data, technology, and privacy to continue evolving in a balanced and sustainable manner, it is necessary to establish international norms and rules. How can we build a system where privacy and technical progress go hand in hand? This must lead to an environment where both can thrive. The story of artificial intelligence, machine learning, and the integration of public online data is still evolving around this topic.
5. Advancements in technology
When privacy, data correctness, and moral worries are big issues, new technologies are not only useful; they’re necessary. To efficiently and responsibly use public online data, these solutions fill in the gaps and overcome the obstacles.
6. Dynamic models and algorithms
Machine learning models and advanced algorithms have progressed to a point where they can now operate with greater subtlety and discernment. The capacity to manage many types of data, differentiate between them, check their accuracy, and ensure adherence to privacy and ethical standards are all strengths of theirs. We need massive amounts of data. That being said, there are also ethical and privacy considerations that must be considered.
7. Validation of data
Technology for data validation also makes sure the data being used is current and reliable. To guarantee that the insights and choices made from this data are reliable and legitimate, we train AI models to detect and remove inaccurate, irrelevant, or out-of-date data. These technological advancements will be crucial as we keep using public web data to power ML and AI applications. Their job is to make sure that the data-harvesting process is sustainable, ethical, and follows all the rules when it comes to privacy and the law in our digital world.
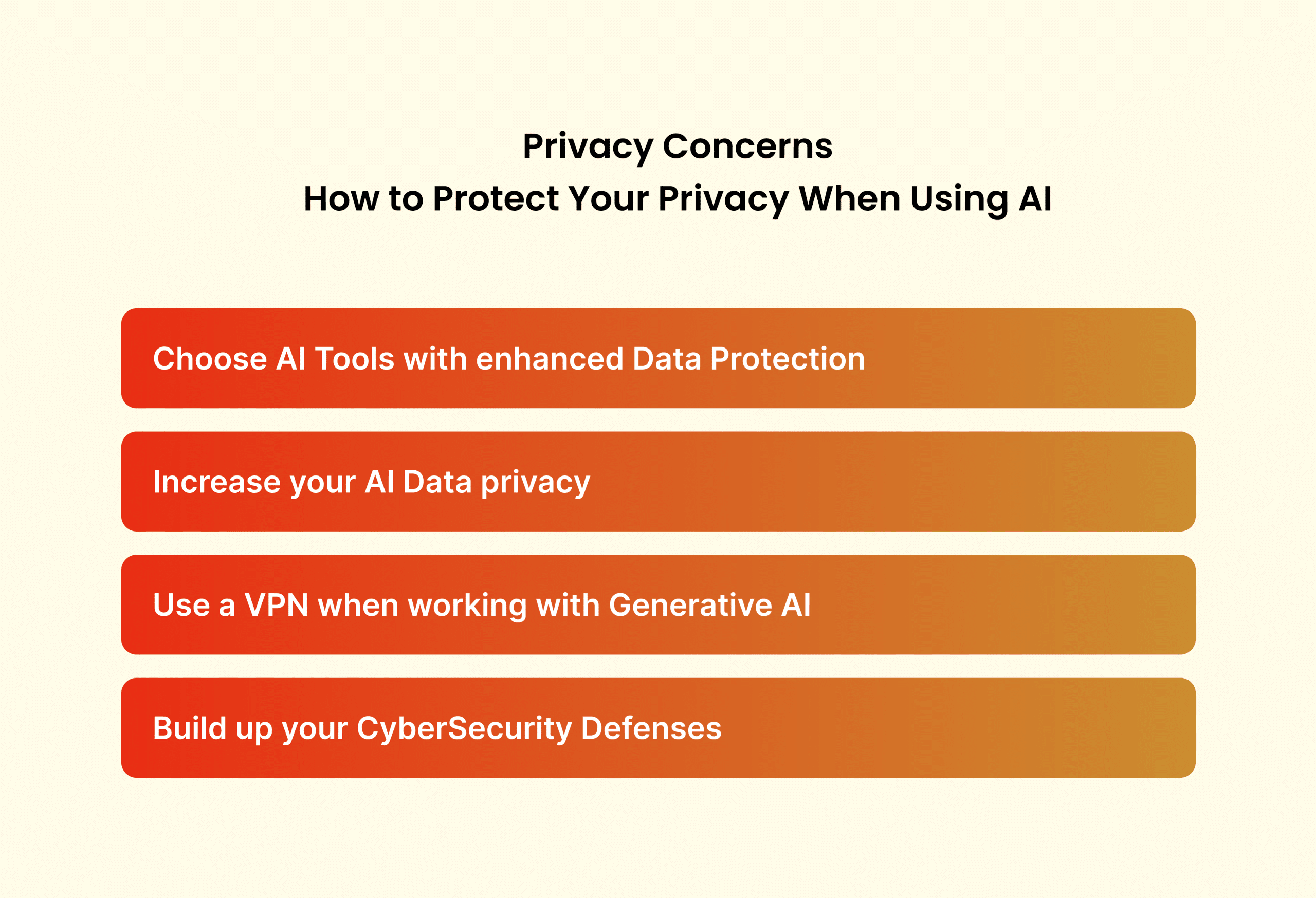
We need to find a way to incorporate and modify these advances so that we can maintain and improve the balance between data use and privacy. Lots of new developments in artificial intelligence, machine learning, and open web data will try to answer this crucial topic.
8. New potential
The combination of public web data, AI, and ML is not only a passing fad. It is a revolutionary force that is changing the face of business, technology, and innovation in the dynamic digital world. Together, these forces will unleash a deluge of data and usher in a new era where information is useful, moral, and crucial in propelling game-changing choices.
9. Analytics for the future
With the use of publicly available online data, AI models can now provide highly accurate and individually tailored forecasts. Predicting future patterns and behaviors with an unprecedented level of accuracy is the goal. We are shifting our focus from broad generalizations to more nuanced, personalized forecasts that take into account individual and market characteristics. Just how will this level of accuracy change the way companies operate and the way customers feel?
10. Progress in innovation
With the rise of AI, ML, and open web data, innovation is becoming a continuous process. In this new paradigm, data-driven, customer-centric strategies firmly rooted in real-time market dynamics supersede traditional paradigms of innovation. From the melting pot of large, varied, and real-time data come innovations. Customizing breakthroughs that resonate, adapt, and develop is more important than making the next big product.
At the dawn of this new age, the convergence of open online data, artificial intelligence, and machine learning beckons us to enter a world where boundaries are not just blurring but being redrawn. Here, data takes on the form of a tale, one that is revealed in real-time and provides insights, influences choices, and creates breakthroughs. All the potential and promise of the future is not some faraway future; it is here and now.